3 Considerations for Configuring your Ecommerce Experiments
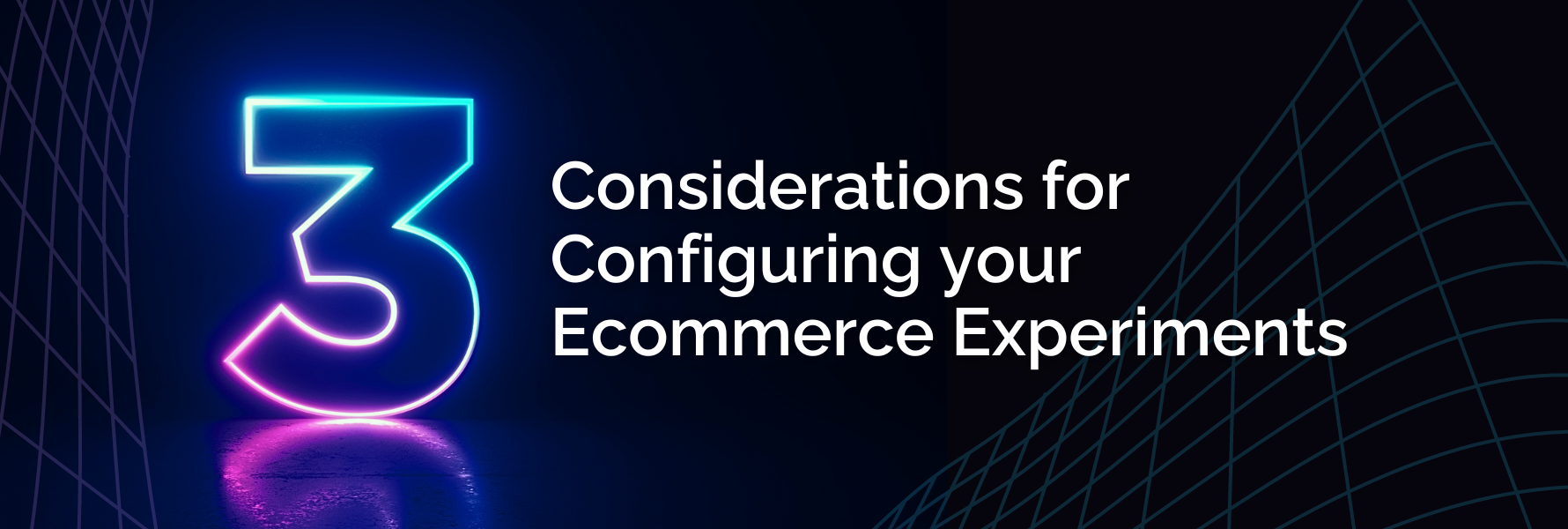
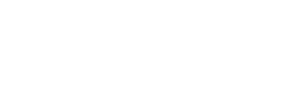
Although we'd argue there is no such thing as a perfect experiment nor a perfect experiment formula (we aren't big supporters of one-size-fits-all templates), we do emphasize the importance of smart testing – fuelled by curiosity, powered by flexibility, aligned for learning, and set up for scalability.
In other words, let your optimization goals run wild and your thoroughness keep you grounded. To help you do exactly that, this blog post outlines three fundamental considerations to incorporate when configuring your next ecommerce experiment.
1. What (exactly) is the goal?
It sounds obvious; without a goal in mind, you probably wouldn't be planning any sort of test, but your goal needs to be deeply understood and fleshed out. Here's how to do exactly that...
For starters, clearly define the metric you're aiming to improve. "Conversion" – although inarguably a fantastic goal – is simply too vague. Specify if it's increasing traffic, sales, clicks, AOV, reducing abandoned carts, etc. Next, get specific. Clarify exactly what change you want to see in the metric you're targeting. For example, note that the purpose of your test is to reduce your abandoned cart rate and you're aiming to get it down to 20%.
But before you lock in this goal, you've got to get into the nitty gritty – starting with some self-reflection. Do some digging to answer these questions:
- What is your current abandoned cart rate?
- Has it recently seen any significant changes? If yes, why might that be?
- Has your brand ever reached and held the specific metric you're aiming for?
Once you've taken a good look at your brand's current and historical standing with regard to the metric your test is aiming to improve, you also need to get to know how you compare to the norm through a process called benchmarking. These questions will deepen your knowledge of standard metrics for brands and industries like yours:
- What is the average/standard abandoned cart rate?
- Is your current abandoned cart rate on par with, notably worse, or better than the industry standard?
Where can you find benchmark data? Dynamic Yield offers a user-friendly overview of some ecommerce industry metrics and Semrush offers a free trial access to their market insights report. But for the most in-depth, routinely-updated benchmarking data, you may want to lean on paid resources like Bizminer or eMarketer. But wherever you source your benchmark data, pay close attention to the dates, industry details, and filter criteria (i.e. region or device type) and be confident it's a reputable source to ensure you're accessing accurate, relevant, and up-to-date information.
And now, unlike many teams who either overlook or give uninformed answers to the final goal-determining questions, you'll be fully equipped to decide:
- Is this specific goal achievable?
- Is it worth the time, effort, resources, and budget to proceed with a test that targets this goal?
If all signs point to "Yes", you've officially got the green light – and all of the insights needed – to make an educated hypothesis. The test results in conjunction with your hypothesis aren't to draw attention to your fortune-telling abilities, but together will make the outcome all the more meaningful and will help you better conduct and hypothesize the results of your future experiments.
2. What will you test to achieve your goal – and how?
So, you've cemented the exact purpose of your test and the specific goal you're aiming to achieve. Now, how are you going to do it? There are many types of experiments, but the most common are A/B or multivariate tests. If that's your chosen route, deciding on the number of variants is just the first step.
You also have to determine whether you're going to conduct a small, incremental test or a larger-scale, rapid-fire test.
The former, in an A/B test format, might look like testing two checkout page variants that have different "Buy Now" button colors, but are otherwise identical – a slight variation that you could later build upon incrementally by, for example, testing two variants with the winning button color, but changing the button text.
These small, slow-build experiments could be best-suited for your team if your prior digging and research led you to believe that one very specific component may result in a significant change. Many teams also opt for these kinds of tests because they lack the bandwidth and resources to create more diverse variants – if slow and steady or no testing at all are their only options.
Larger-scale, rapid-fire testing, in an A/B experiment format, might look like creating and comparing two different checkout page variants with different layouts, button colors, and copy – an entirely different experience for the traffic assigned to each.
These more expansive variant types are the best experiment fuel when there isn't an obvious, specific component you've determined is holding you back from having already achieved your goal or if you want to gain more extensive insights from your testing efforts. Most likely to opt for these kinds of tests are teams with the bandwidth, resources, and tools to create highly differentiated variants and experiment at scale.
With the number and type of variants decided, you can move on to determining your experiment structure which might include things like sample size, timing, and duration. This is all about finding a balance between good, representative data (AKA statistical confidence) and getting results fast.
One key consideration: your brand calendar. This will help you determine when to run your test as well as any foreseeable factors that might affect your results. However, keep in mind that occurrences like price changes, promos, or seasonal campaigns could either help or hinder your tests. For example, if you're testing two different hero images on your home page and comparing click rates, then anything expected to drive higher-than-usual traffic might be beneficial as you may be able to harness more data faster. However, if the goal is to improve AOV and you're testing a product recommendation carousel during a sales campaign where your prices are reduced, your carousel's effect on your AOV metric might be skewed.
Unsurprisingly, experimentation is often associated with science which paves the way for a variety of left-brained style "best practices" for mapping out the perfect experiment structure. Some of these emphasize the importance of a minimum 1-week test duration, relying on test sample size calculators, or using rigorous formulas to determine statistical confidence...
...But here's our hot take:
If your team has the tools that provide flexibility, scalability, and speed in creating variants and running experiments, then do your due diligence (i.e. address the considerations outlined in this post), but don't get caught up in the science and math of experimentation or get stalled by months-long wait times for your variants to be created and your tests to run. Your experiments don't have to be perfect if your team is able to monitor active tests, recognize directional data indicators, make quick decisions, and continue to iterate and test.
3. What do you plan to do with the results?
Data and data analysis go hand in hand, but don't fret, this doesn't have to be a math or a science either. You have a well thought-out goal in mind, so what's the equally well thought-out plan once the results are in?
The first thing you're going to look at, naturally, is if your experiment moved the needle on the metric you were aiming to affect. Be prepared to compare the results against your hypothesis and also revisit seemingly extraneous factors that may have been at play. You already looked at and accounted for foreseeable variables, but in hindsight, you may be able to pinpoint different or unexpected impacting factors such as price changes, unusual traffic sources, or website performance issues that occurred.
Remember that data is good, but actionable data reigns supreme, so ask yourself a few questions in advance to determine what action you might take depending on what results your test yields:
- What results would you consider sufficient directional indications?
- Will you make changes to your site by implementing your winning variant?
- Will you run the exact same test again? When?
- Or will you iterate your test and compare different variants?
And to keep the experimentation ball rolling, think about and start tackling any prep work you can do now to support and speed up your next test – be it an iteration of this one or something entirely different.
Another important note: Although you've taken the time to consider and clarify your specific goals, don't hyper-focus on the metric you set out to improve. Remove the blinders and be prepared to look at all data from your experiment; there may be something to learn from your test that you weren't expecting.
And consider this...
We talk about not getting caught up in or slowed down by the math, science, and rigor often associated with ecommerce experimentation. The key is balancing the creativity and curiosity that fuels your testing efforts with the processes that make your efforts worthwhile. The considerations outlined in this post along with organized documentation of your goals, current metrics, plan, quantitative results, and qualitative observations will enable you to test smart, not hard.
And if you have the thirst to test more and faster, but your lack of dev resources and experiment-enabling tech is standing in the way, here's one more consideration: Why settle for a snail-like pace if it's possible to bypass dev handoffs and wait times and take control of your ecommerce site – including the content that powers scaled experimentation? Explore Fastr Frontend and imagine your brand's limitless potential if your ecommerce team was equipped with that level of flexibility, creative freedom, and agility.